Summary by Haoyu Wang
In today’s rapidly evolving field of Natural Language Processing (NLP), the quest for achieving deeper semantic understanding of texts continues to accelerate. In this new paper, “Event Semantic Classification in Context,” we demonstrate how classifying events from multiple perspectives can greatly enhance machines’ ability to understand and reason about events.
Understanding the Complex Realm of Event Semantics
Instead of the broad-brush approach of classifying easily understandable lexical items such as nouns, we delve into the nuanced domain of events. Events in texts are not mere occurrences; they are the pivot around which a narrative’s temporal dynamic, causality, and thematic progression revolve. This research classifies events based on six properties: modality, affirmation, specificity, telicity, durativity, and kinesis.
The Six Essential Properties for Event Classification:
- Modality (Actuality) – Determines whether an event actually takes place.
- Affirmation – Indicates whether an event is described affirmatively or negatively.
- Specificity (Genericity) – Ascertains whether an event is a singular occurrence or part of a general trend.
- Telicity (Lexical Aspect) – Identifies whether an event has a definite end.
- Durativity (Punctuality) – Determines the duration over which an event unfolds.
- Kinesis – Differentiates between states and actions.
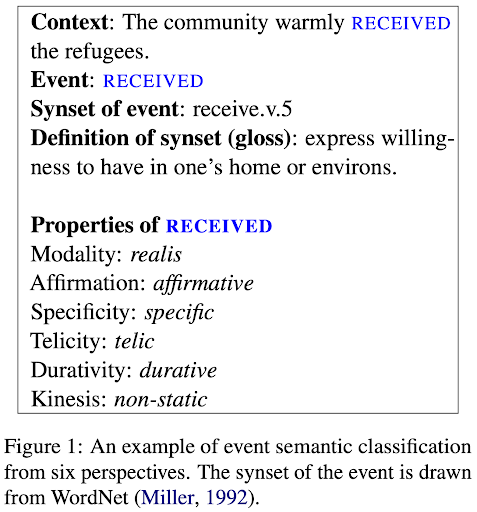
The significance of these classifications extends beyond mere semantic labeling. They provide foundational insights into how events are grounded in time and reality, laying the groundwork for more refined event understanding and reasoning—a leap forward in machine comprehension of narratives.
The ESC Dataset
One of the main contributions of this work is the introduction of the ESC (Event Semantic Classification) dataset. This novel bilingual dataset, encompassing both English and Chinese, is specifically crafted for fine-grained semantic classification tasks. It stands out for its inclusion of all example sentences from WordNet featuring frequent verbs, tagged with six aforementioned semantic properties concerning events.
Still Challenging for ChatGPT
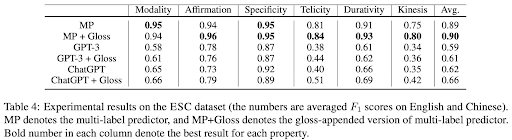
We find that these fine-grained semantic understanding tasks are challenging for ChatGPT, while they can be well solved by fine-tuning smaller language models like XLM-RoBERTa.
Advancing Event Understanding and Reasoning
By integrating the classification of events according to these detailed semantic properties, the research demonstrates a marked improvement in event understanding and reasoning capabilities. This is meticulously evidenced through experiments focusing on tasks such as event extraction, temporal relation extraction, and subevent relation extraction. Notably, the dataset and the sophisticated classification models designed in this study are instrumental in making substantive advancements in these areas. By leveraging innovative datasets like ESC and pushing the boundaries of event classification, the NLP field is inching closer to unlocking the full potential of machines in understanding the intricacies of human language and thought.
To read the full paper: Haoyu Wang, Hongming Zhang, Kaiqiang Song, Dong Yu, and Dan Roth, Event Semantic Classification in Context, Findings of EACL (2024).
Dataset forthcoming.
Haoyu Wang is a third-year PhD student in the Cognitive Computation Group at the University of Pennsylvania, with a research interest in event-centric natural language understanding.