The 2024 Conference of the North American Chapter of the Association for Computational Linguistics (NAACL) is coming up, from June 16-21 in Mexico City. We’re excited to share the work that’s being presented and published from CCG and our collaborating authors. You can find links to our NAACL papers below!
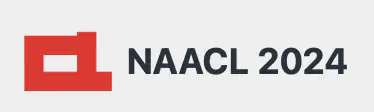
Sub-Sentence Encoder: Contrastive Learning of Propositional Semantic Representations
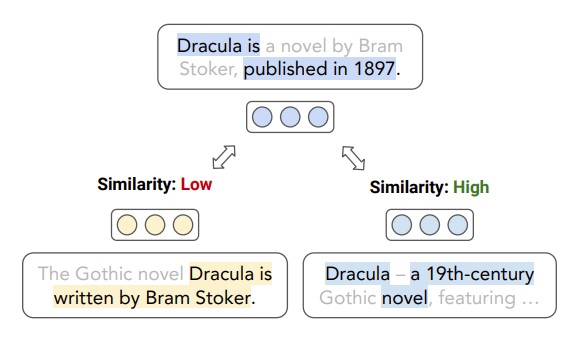
Text embeddings typically produce one embedding for the entire text sequence, but what if the text is long and says many things? Check out Sub-Sentence Encoder — A contextual text encoder model that learns to embed individual pieces of meaning in text.
Sihao Chen, Hongming Zhang, Tong Chen, Ben Zhou, Wenhao Yu, Dian Yu, Baolin Peng, Hongwei Wang, Dan Roth, and Dong Yu, Sub-Sentence Encoder: Contrastive Learning of Propositional Semantic Representations (NAACL 2024).
SocREval: Large Language Models with the Socratic Method for
Reference-Free Reasoning Evaluation
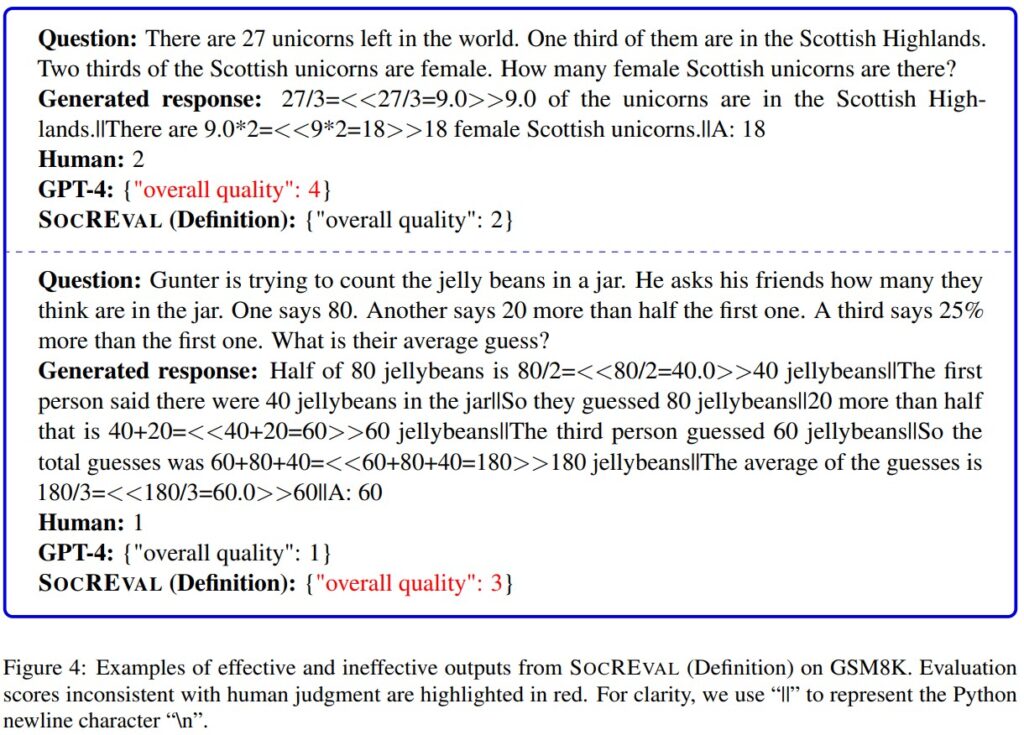
The paper develops a reference-free evaluation of reasoning abilities of LLMs, surpassing the abilities of GPT-4 to evaluate reasoning abilities.
Hangfeng He, Hongming Zhang, and Dan Roth, SocREval: Large Language Models with the Socratic Method for Reference-free Reasoning Evaluation (NAACL Findings 2024).
What if you said that differently?: How Explanation Formats Affect Human Feedback Efficacy and User Perception
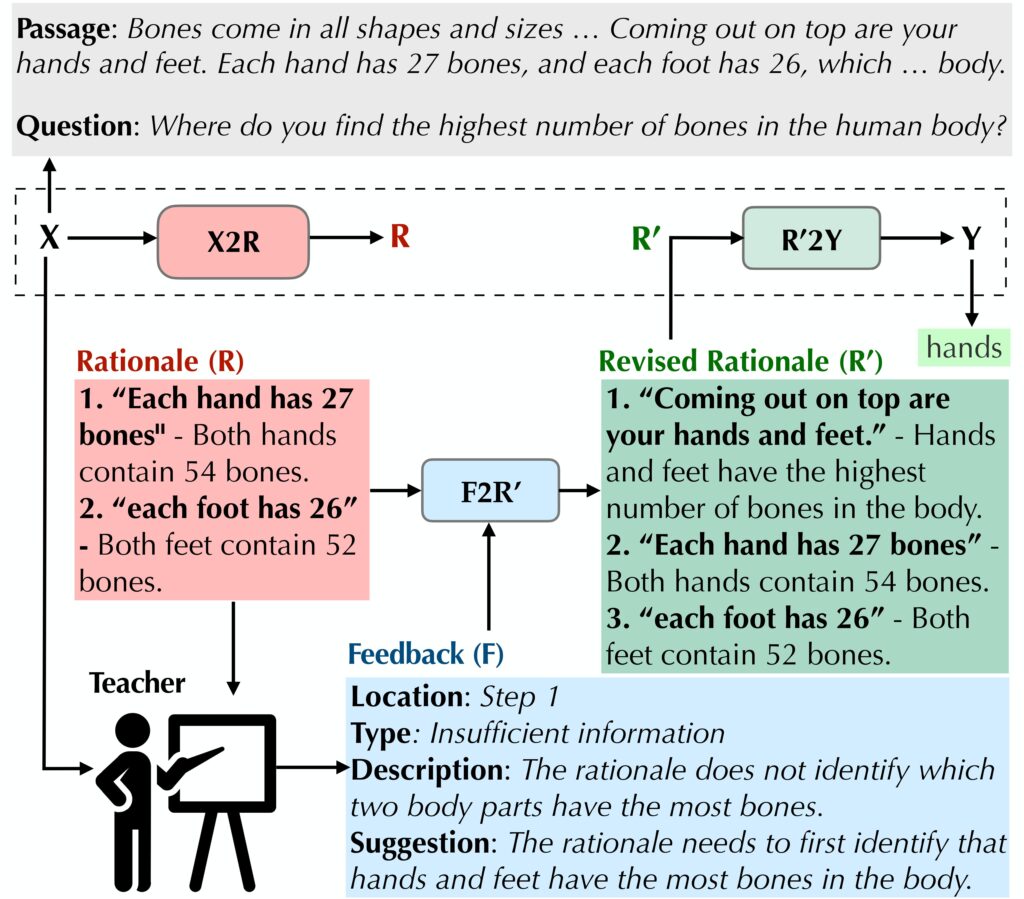
In this work, we study the effect of intermediate explanation formats on the effectiveness of human feedback for correcting QA model responses. Further, we investigate the properties of explanations which allow users to understand and trust responses.
Chaitanya Malaviya, Subin Lee, Dan Roth, and Mark Yatskar, What if you said that differently? How Explanation Formats Affect Human Feedback Efficacy and User Perception (NAACL 2024).
ExpertQA: Expert-Curated Questions and Attributed Answers
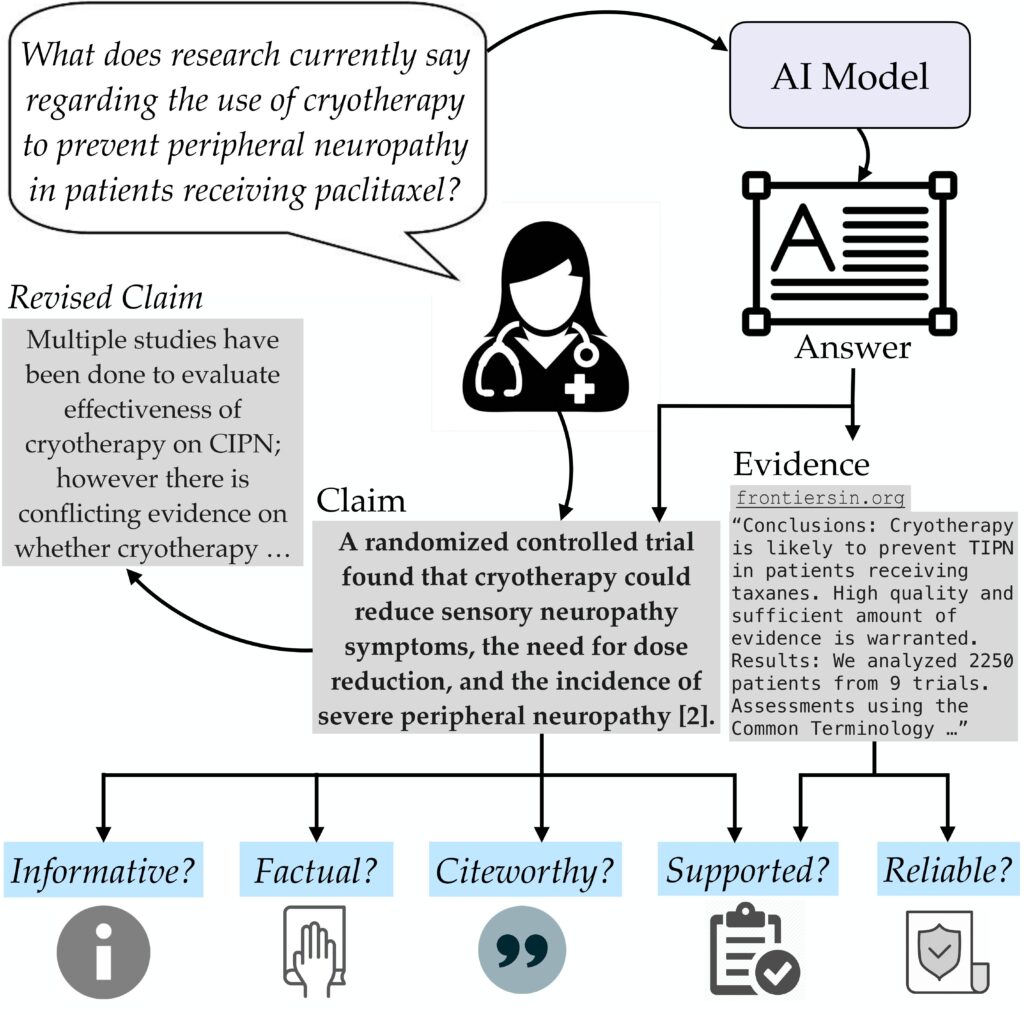
This work conducts expert evaluation of responses to domain-specific questions according to various axes of attribution and factuality. Based on our evaluation, we present ExpertQA, a high-quality long-form QA dataset with 2177 questions spanning 32 fields, along with verified answers and attributions for claims in the answers.
Chaitanya Malaviya, Subin Lee, Sihao Chen, Elizabeth Sieber, Mark Yatskar, and Dan Roth, ExpertQA: Expert-Curated Questions and Attributed Answers (NAACL 2024).
ReEval: Automatic Hallucination Evaluation for Retrieval-Augmented
Large Language Models via Transferable Adversarial Attacks
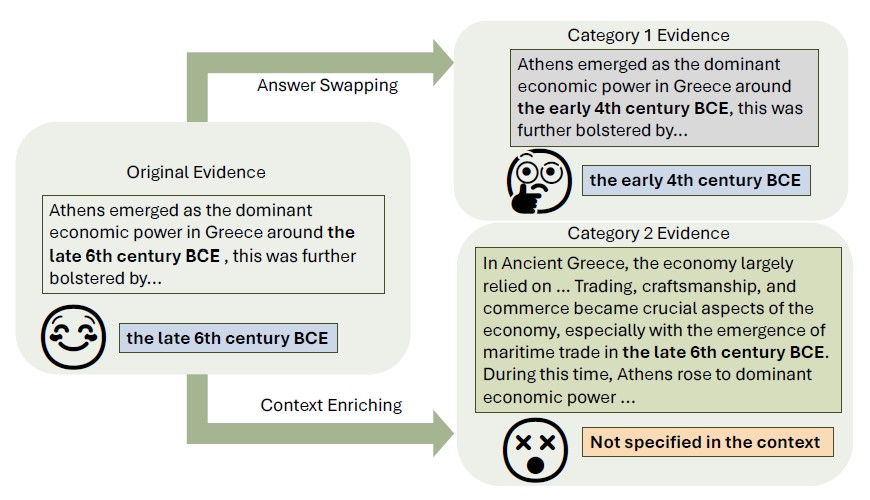
Despite remarkable advancements in mitigating hallucinations in large language models (LLMs) by retrieval augmentation, it remains challenging to measure the reliability of LLMs using static question-answering (QA) data. Inspired by adversarial machine learning, we investigate the feasibility of automatically perturbing existing static benchmarks for dynamic evaluation. Specifically, this paper presents ReEval, an LLM-based framework using prompt chaining to perturb the original evidence and generate new test cases for evaluating the LLMs’ reliability in using new evidence for answering.
Xiaodong Yu, Hao Cheng, Xiaodong Liu, Dan Roth, Jianfeng Gao, ReEval: Automatic Hallucination Evaluation for Retrieval-Augmented Large Language Models via Transferable Adversarial Attacks (NAACL Findings 2024).