The 2025 Conference of the Nations of the Americas* Chapter of the Association for Computational Linguistics (NAACL) will be coming up in May, in Albuquerque, New Mexico. We’re excited to share the work that will be presented and published by the group and our collaborating authors. You can find links to our NAACL 2025 papers below!
___
* Here’s a blog post from the NAACL organizing committee about their name change.
On Reference (In-)Determinacy in Natural Language Inference
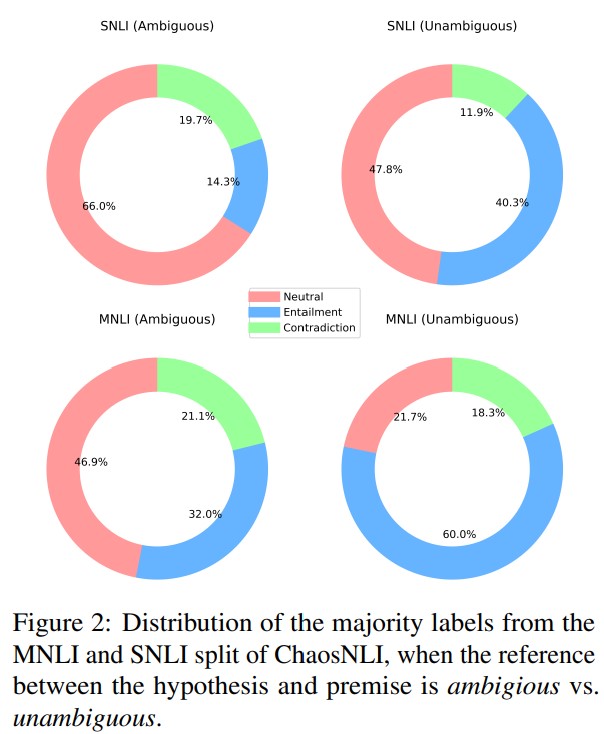
This paper introduces RefNLI, a diagnostic benchmark for identifying reference ambiguity in Natural Language Inference examples. We provide insight into how the reference determinacy assumption (the assumption that premise and hypothesis refer to the same context) impacts the downstream utility of NLI models, and discover that the existence of reference ambiguity in NLI examples can in part explain the inherent human disagreements in NLI.
Sihao Chen, Chaitanya Malaviya, Alex Fabrikant, Hagai Taitelbaum, Tal Schuster, Senaka Buthpitiya, and Dan Roth, On Reference (In-)Determinacy in Natural Language Inference. NAACL Findings (2025)
Towards Long Context Hallucination Detection
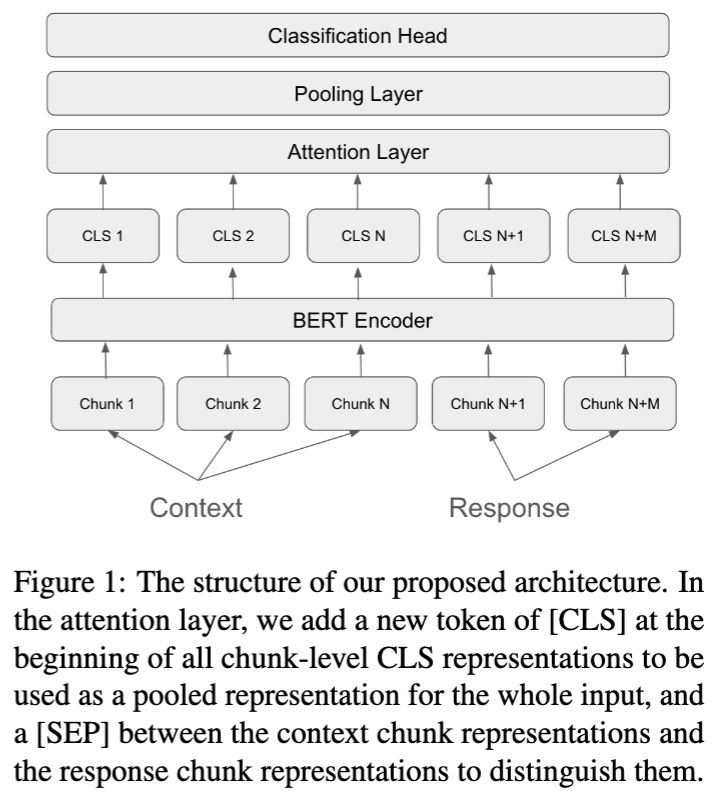
This paper studies hallucination detection where the context length is long (>=512 tokens). We construct a dataset to evaluate the task and propose a method to approach it.
Siyi Liu, Kishaloy Halder, Zheng Qi, Wei Xiao, Nikolaos Pappas, Phu Mon Htut, Neha Anna John, Yassine Benajiba, and Dan Roth, Towards Long Context Hallucination Detection. NAACL Findings (2025)
Open Domain Question Answering with Conflicting Contexts
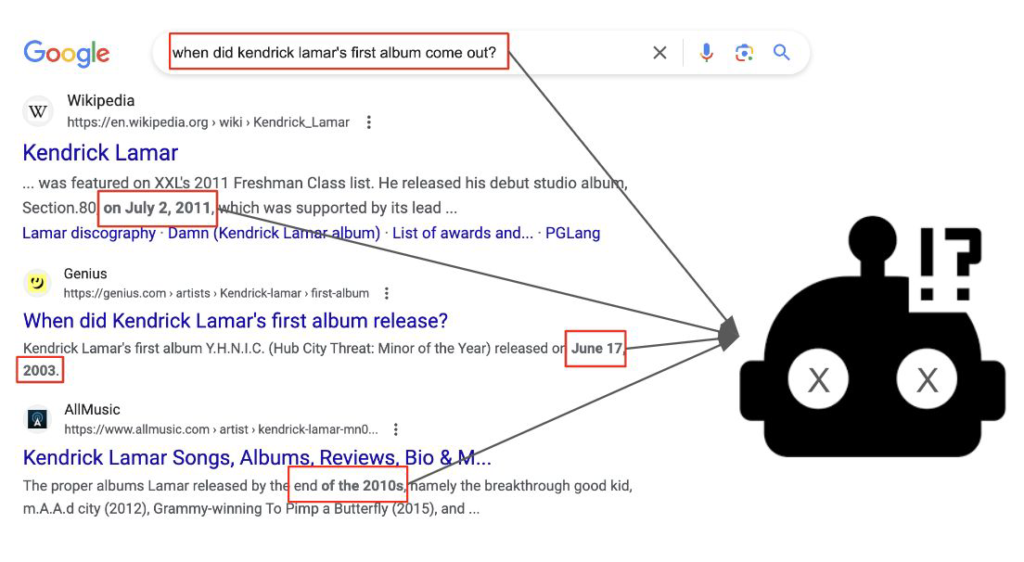
We study open domain question answering when there is conflicting evidence presented on the web. We demonstrate that by finetuning LLMs to explain their answers, we can introduce richer information into their training that guides them through the process of reasoning with conflicting contexts.
Siyi Liu, Qiang Ning, Kishaloy Halder, Zheng Qi, Wei Xiao, Phu Mon Htut, Yi Zhang, Neha Anna John, Bonan Min, Yassine Benajiba, and Dan Roth, Open Domain Question Answering with Conflicting Contexts. NAACL Findings (2025)
Hallucination Detection. NAACL Findings (2025)
H-STAR: LLM-driven Hybrid SQL-Text Adaptive Reasoning on Tables
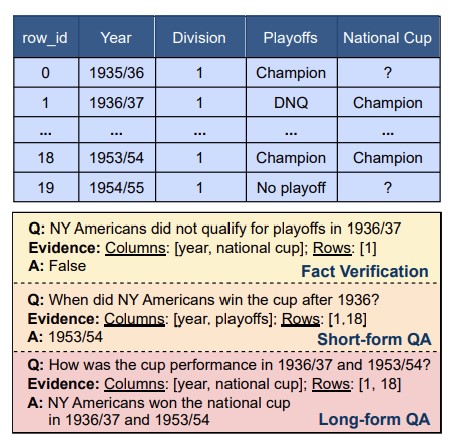
Existing approaches to tabular reasoning employ either textual reasoning, which excels in semantic interpretation but struggles with mathematical operations, or symbolic reasoning, which handles computations well but lacks semantic understanding. H-STAR, a novel method introduced in this paper, integrates text comprehension with SQL-like logic to effectively answer queries from structured tables.
Nikhil Abhyankar, Vivek Gupta, Dan Roth, and Chandan K. Reddy, H-STAR: LLM-driven Hybrid SQL-Text Adaptive Reasoning on Tables. NAACL (2025)
TRANSIENTTABLES: Evaluating LLMs’ Reasoning on Temporally Evolving Semi-structured Tables
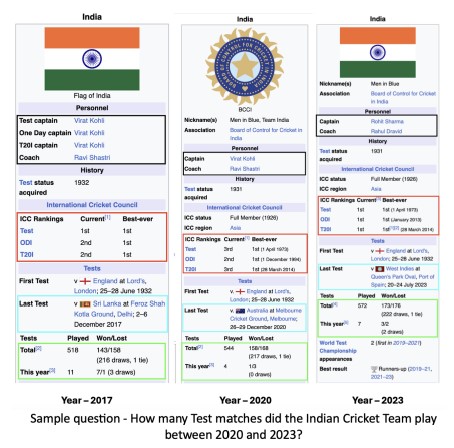
The ability to reason over time allows us to identify future steps and to understand the effects of decisions on our lives. However, large language models are typically trained on static datasets, limiting their ability to perform effective temporal reasoning. To assess the temporal reasoning capabilities of LLMs, this paper presents the TRANSIENTTABLES dataset, with questions derived from over 14,000 tables, spanning multiple time periods.
Abhilash Shankarampeta, Harsh Mahajan, Tushar Kataria, Dan Roth, and Vivek Gupta, TRANSIENTTABLES: Evaluating LLMs’ Reasoning on Temporally Evolving Semi-structured Tables. NAACL (2025)
Enhancing Temporal Understanding in LLMs for Semi-structured Tables
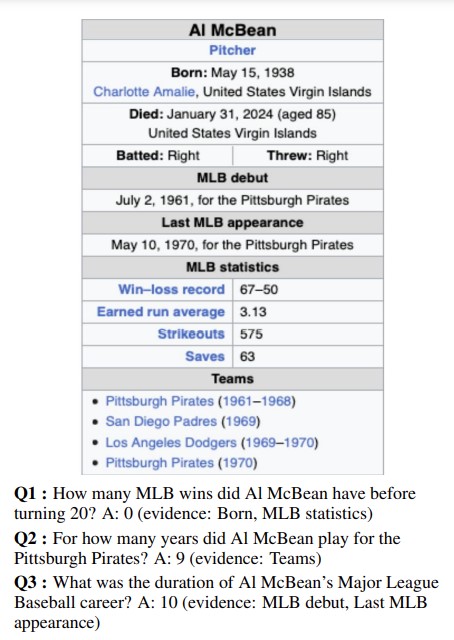
We introduce the C.L.E.A.R. prompting framework and auxiliary cross-format training to enhance LLM performance in temporal tabular reasoning. Our findings demonstrate that our method improves evidence-based reasoning across various models. Additionally, our experimental results reveal that indirect supervision with auxiliary unstructured data (TRAM) substantially boosts model performance.
Irwin Deng, Kushagra Dixit, Vivek Gupta, and Dan Roth, Enhancing Temporal Understanding in LLMs for Semi-structured Tables. NAACL Findings (2025)
MAPWise: Vision-Language Models for Advanced Map Queries
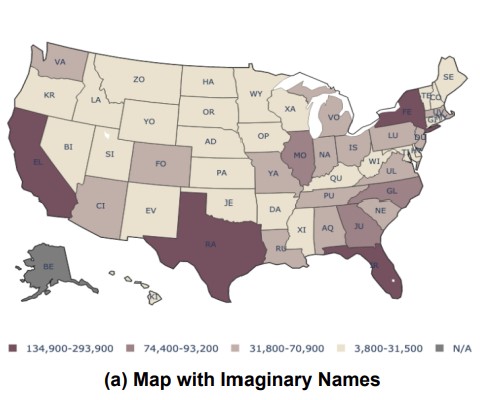
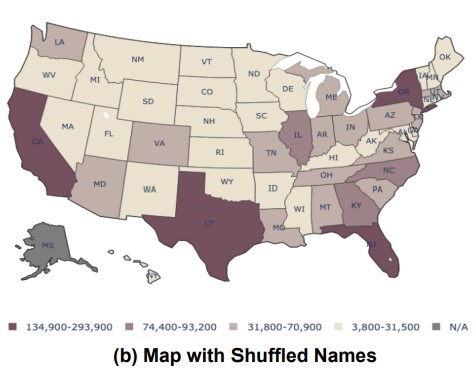
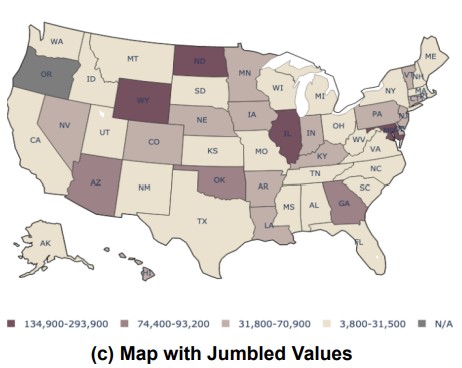
This paper introduces a new benchmark for evaluating vision-language models (VLMs) on choropleth map question answering, featuring diverse maps and question types across multiple geographic regions. Evaluation of several VLMs reveals significant performance gaps, highlighting the need for further research in this area and providing a resource for future model development.
Srija Mukhopadhyay, Abhishek Rajgaria, Prerana Khatiwada, Manish Shrivastava, Dan Roth, and Vivek Gupta, MAPWise: Evaluating Vision-Language Models for Advanced Map Queries. NAACL (2025)
NTSEBENCH: Cognitive Reasoning Benchmark for Vision Language Models
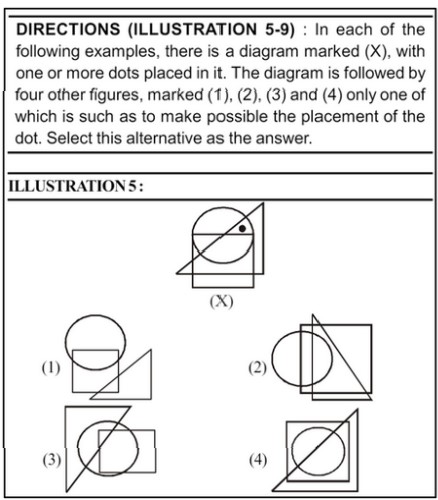
NTSEBENCH is a Vision-Language Model benchmark dataset with 2,728 questions and 4,642 images from India’s NTSE exam, to evaluate VLMs on cognitive multimodal reasoning.
Pranshu Pandya, Vatsal Gupta, Agney Talwar, Tushar Kataria, Dan Roth, and Vivek Gupta, NTSEBENCH: Cognitive Reasoning Benchmark for Vision Language Models. NAACL Findings (2025)
Aligning to Constraints for Data-Efficient Language Model Customization
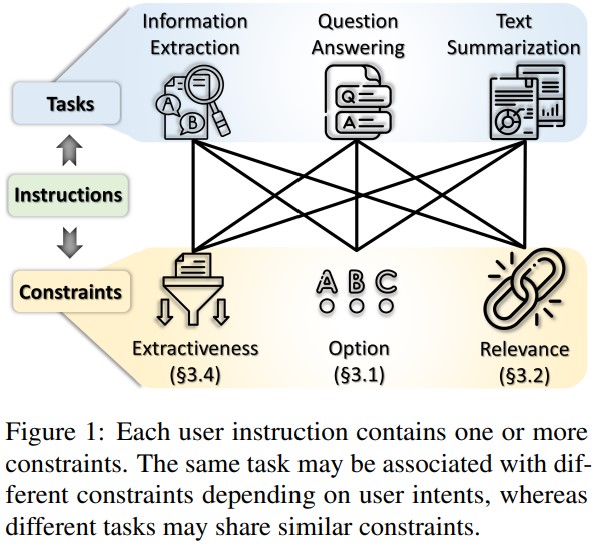
This paper proposes ACT (Aligning to ConsTraints), a unified and efficient Language Model customization framework using automatic constraint verifiers to provide supervision signals for adapting models to downstream tasks.
Fei Wang, Chao Shang, Sarthak Jain, Shuai Wang, Qiang Ning, Bonan Min, Vittorio Castelli, Yassine Benajiba, and Dan Roth, Aligning to Constraints for Data-Efficient Language Model Customization. NAACL Findings (2025)
Leveraging LLM For Synchronizing Information Across Multilingual Tables

We explored the application of large language models (LLMs) for multilingual information synchronization, focusing on improving the accuracy and coherence of updates to Wikipedia tables in low-resource languages.
Siddharth Khincha, Tushar Kataria, Ankita Anand, Dan Roth, and Vivek Gupta, Leveraging LLM For Synchronizing Information Across Multilingual Tables. NAACL (2025)